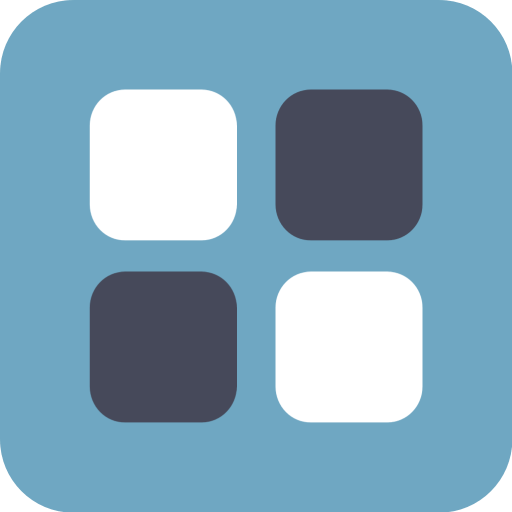
About Confusion Matrix Calculator
Confusion Matrix Calculator: A Comprehensive Tool for Classification Model Evaluation
The Confusion Matrix Calculator is an essential tool designed to evaluate the performance of classification models by calculating various statistical measures derived from the confusion matrix. This application serves as a robust solution for professionals working with machine learning, data science, or any field requiring the assessment of classification algorithms. By leveraging the power of the confusion matrix, this calculator provides insights into the effectiveness of models in predicting outcomes accurately.
At its core, the confusion matrix represents the foundation for understanding model performance. It organizes predictions into four key categories: True Positives (TP), True Negatives (TN), False Positives (FP), and False Negatives (FN). These metrics form the basis for determining critical evaluation metrics such as Sensitivity, Specificity, Positive Predictive Value (PPV), Negative Predictive Value (NPV), False Positive Rate (FPR), False Discovery Rate (FDR), False Negative Rate (FNR), Accuracy (ACC), and Matthews Correlation Coefficient (MCC).
Sensitivity, often referred to as Recall or True Positive Rate (TPR), quantifies the proportion of actual positive cases correctly identified by the model. This metric is crucial for ensuring that the model does not overlook significant events. Conversely, Specificity, or True Negative Rate (TNR), assesses the model's ability to identify actual negative cases. These two measures together provide a balanced view of the model's predictive capability across both positive and negative classes.
Additional metrics like PPV (Precision) and NPV offer further granularity in evaluating model reliability. PPV calculates the proportion of positive predictions that are indeed correct, while NPV evaluates the proportion of negative predictions that are accurate. Together, these metrics help refine the model's ability to minimize false alarms and missed detections.
The False Positive Rate (FPR) and False Discovery Rate (FDR) serve as complementary measures to ensure the model avoids unnecessary errors. FPR identifies the ratio of false alarms among all actual negatives, while FDR highlights the proportion of incorrect positive predictions among all positive predictions. Similarly, the False Negative Rate (FNR) evaluates the model's tendency to miss actual positive cases, providing a comprehensive assessment of potential underpredictions.
Accuracy (ACC) remains a widely recognized metric, representing the overall correctness of the model's predictions. However, the F1 Score adds depth by combining Precision and Recall into a single value, emphasizing the balance between false positives and false negatives. Finally, the Matthews Correlation Coefficient (MCC) offers a nuanced perspective by accounting for all four quadrants of the confusion matrix, providing a score ranging from -1 to +1 that reflects the model's consistency and reliability.
In summary, the Confusion Matrix Calculator is an indispensable resource for anyone seeking to evaluate classification models rigorously. By computing these diverse metrics, users gain actionable insights into their models' strengths and weaknesses, enabling informed decisions and iterative improvements. Whether you're a researcher, developer, or practitioner, this tool empowers you to refine your models and achieve superior performance in classification tasks.
Confusion Matrix Calculator Screenshots
Old Versions of Confusion Matrix Calculator
Confusion Matrix Calculator FAQ
How do I download Confusion Matrix Calculator from PGYER APK HUB?
Is the Confusion Matrix Calculator on PGYER APK HUB free to download?
Do I need an account to download Confusion Matrix Calculator from PGYER APK HUB?
How can I report a problem with Confusion Matrix Calculator on PGYER APK HUB?
User Reviews
+ Reviews
Most Popular























